Here is an example of research conducted in our laboratory.
“Exploring Interlocutor Gaze Interactions in Conversations based on Functional Spectrum Analysis”
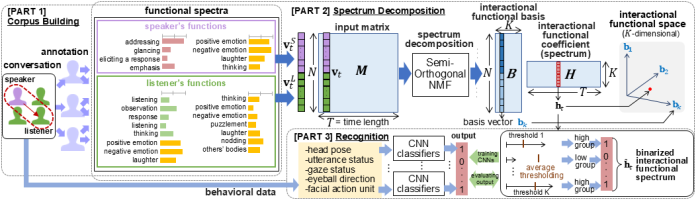
【Summary】
Interlocutors’ gaze behaviors play essential roles in dialogue, such as observing others and adjusting the flow of conversation. Considering the importance of gaze behaviors, we defined 43 gaze functions to analyze and recognize them. As a part of this research, we proposed a novel framework named a gaze interactional functional spectrum analysis (GI-FSA) to explore the functional aspects of gaze interactions between the speakers and listeners in conversations. This study introduces a novel representation called a gaze functional spectrum to capture the intrinsic nature of gaze functions, such as multiple functionalities and ambiguity in its interpretation. The gaze functional spectrum represents the distribution of perceptual intensities of multiple gaze functions, which are calculated as the percentages of raters who found each function to be present. Then, to reveal the primary and distinctive interactional functionalities that emerge via the gaze behavior, spectrum decomposition is performed on a matrix of concatenated vectors of the functional spectra of both speakers and listeners over time. We have proposed a semiorthogonal nonnegative matrix factorization as a method for this spectrum decomposition. This matrix factorization decomposes the input matrix into a product of a basis matrix and a coefficient matrix. The basis matrix represents primary and distinctive gaze interactional functions. The coefficient matrix represents the temporal activation of gaze interactional functions. This spectrum decomposition projects the functional spectra from interacting speakers and listeners into a lower dimensional space. We named this projection as the “interactional functional spectrum.” In addition, we proposed a convolutional neural network (CNN) that recognizes the interactional functional spectrum from the observable nonverbal behaviors of the interlocutors. Experimental results on the proposed method suggest that GI-FSA is a promising research framework for analyzing and understanding interlocutor gaze interactions.
【Bibliography】
ICMI
Ayane Tashiro, Mai Imamura, Shiro Kumano, and Kazuhiro Otsuka, “Exploring Interlocutor Gaze Interactions in Conversations based on Functional Spectrum Analysis”, 26th ACM International Conference on Multimodal Interaction (ICMI2024), pp.86-94, Presented in November 2024.
Conference Paper URL:https://doi.org/10.1145/3678957.3685708
電子情報通信学会HCGシンポジウム
田代絢子, 田嶋桃佳, 熊野史朗, 大塚和弘, 「対話者の視線行動を対象とした相互作用機能スペクトラムの分析」, 電子情報通信学会HCGシンポジウム2024, Presented in December 12, 2024.
Recipient of the Outstanding Interactive Presentation Award
Presentation Program URL:
“Exploring Multimodal Nonverbal Functional Features for Predicting the Subjective Impressions of Interlocutors”
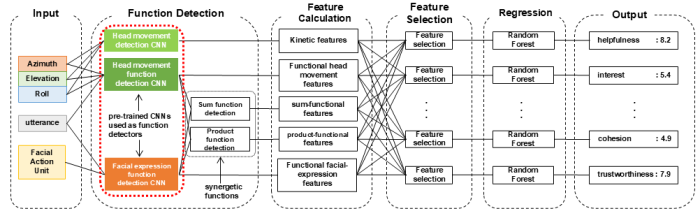
【Summary】
Group discussions take place in various situations, such as decision-making and problem-solving, and the subjective impressions of the interlocutors, including satisfaction and concentration, are closely related to the quality of the discussion. Understanding these subjective impressions can help improve discussion quality by providing appropriate support to the facilitator, who is responsible for facilitating the discussion, and by providing post-discussion feedback. To predict such subjective impressions, we propose a model that utilizes features related to the communicative functions of nonverbal behaviors such as head movements and facial expressions expressed during discussion. The model employs a convolutional neural network (CNN) to detect participants’ head movements and facial expression functions during discussion and defines functional features such as occurrence rate, based on the detection results. Furthermore, sequential feature selection is applied to identify the optimal combination of features, and subjective impression prediction is performed using random forest regression. For the prediction target, we use self-reported scores on a 9-point scale for 16 impression items, such as enjoyment and difficulty, assessed after the discussion by each participant from 17 discussion groups, each consisting of four women. The experimental results showed that predictions made using the proposed method significantly correlated with the self-reported scores for more than 70% of the impression items, which indicates the effectiveness of multimodal nonverbal functional features in predicting subjective impressions.
【Bibliography】
IEEE access
Ito, Koya, Yoko Ishii, Ryo Ishii, Shin-ichiro Eitoku and Kazuhiro Otsuka. “Exploring Multimodal Nonverbal Functional Features for Predicting the Subjective Impressions of Interlocutors,” IEEE Access, Vol.12, pp. 96769-96782, 2024.